Some organizations have been finding ways to implement AI in their data analysis process to save time, improve data collection and quality, and make better decisions.
In this article, I’ll review everything about AI use in data analysis: use cases, benefits, and examples of companies harnessing the power of AI for data analysis.
TL;DR
- The best use cases for AI in data analysis are data collection & cleaning, being able to analyze data sets from sheets, visualizing data, and data enrichment.
- The main benefits of using AI for data analysis include saving time from manual data entry, being able to enrich existing data sets, and making predictions.
- A popular example of organizations using artificial intelligence in their data analysis is Amazon, which uses AI’s predictive capabilities to analyze their customers and recommend products.
- The best tools for AI data analysis are Team-GPT, Luzmo, and Tableau due to their ability to analyze large amounts of data.
Table of contents
How Is AI Used For Data Analysis?
Artificial intelligence is being used for data analysis to:
- Sift through large volumes of customer data.
- Identify patterns in large data sets.
- Generate unique insights.
- Make predictions based on historical and collected data.
- Recommend products based on consumer data.
AI uses machine learning algorithms and deep learning to extract patterns from data, make predictions and get insights from unstructured data.
For example, you can feed your data to a Custom GPT model that can analyze it for you and extract results.
💡 Later in this guide, we’ll explore the different use cases and some examples of organizations that have successfully utilized the technology for predictive analytics and data segmentation.
What Are The Benefits of Using AI for Data Analysis?
The main benefits of using AI for data analysis include being able to save time from manual data entry, enriching existing data sets, and making predictions.
Let’s go over each one of them 👇 in detail.
#1: Save Time From Manual Data Entry & Analysis
The best use case of using AI for data analysis for me has been how it can analyze large chunks of data faster than data scientists.
➡️ Instead of wasting time and money, your data scientists can spend more time on higher-value tasks and analysis, instead of manual data cleaning or processing.
AI can automate repetitive tasks, such as collecting data and then filling it.
#2: Improve Your Existing Data Sets
The next benefit for me of AI has been enriching existing data sets.
Artificial intelligence can enrich existing data sets by augmenting them with relevant external information, which enhances the overall quality and context of your data.
💡 Your brand can improve the accuracy and reliability of insights that you got from the data so you can make informed decisions and deeper analysis across various applications.
#3: Forecast & Predict
Last but not least, AI can be used to make forecasts and predictions off the data that you have collected.
For example, in e-commerce, AI can be used to collect information about your customers and then offer product recommendations.
➡️ In other industries, such as manufacturing, AI can predict when machines could malfunction based on their performances.
6 AI Use Cases for Data Analysis
Here are the 6 best use cases for data analysis from the ones I’ve seen work best:
#1: Data Collection & Cleaning
AI helps you extract data from various data sources that you can feed your AI algorithm, instead of manually inserting and cleaning them.
If your data is cleaner, then your insights are more likely to be valuable and then used for business decisions.
➡️ For example, tools like Team-GPT (our tool) can spot and correct typos, data inconsistencies, and missing values from your data sets.
Your brand can pinpoint outliers in your data to find errors in the data or some special cases, such as wrong input from customers.
#2: Analyzing Data Sets
After you’ve collected your data and cleaned it, organizations can start analyzing the data to receive insights to act upon.
Generative AI tools like ChatGPT, Claude and Perplexity can identify patterns, correlations, anomalies, and overall trends in your data sets.
To scale the process, you can feed them entire spreadsheets and large-size documents that they can then analyze for you.
After the tools have analyzed that data, you can ask them questions related to the data.
💡 For example, you can ask the tools ‘’How much organic traffic did we receive in October 2024 in comparison to October 2023?’’
When it comes to e-commerce, AI can analyze large consumer and commercial data sets to forecast customer demand for products to help you manage their inventories.
The technology analyzes data from inventory levels, shipping routes, and seasonal data to help corporations improve operational efficiency and streamline their supply chains.
#3: Improved Decision-Making
After the AI tools have analyzed your data, you can use them to make lighting speed decisions that take into account the data sets.
For example, with Team-GPT, you can investigate complex data sets and reveal underlying structures to identify trends and correlations.
Alternatively, you can leverage AI to alert you when an action has been triggered, which is a practice used in inventory management.
#4: Predictive Analytics
My favourite use case for data analysis is its predictive analytics which can be used for product recommendations.
The AI-powered platforms are basing their recommendations on historical data that has been collected about your customers or B2B clients.
The technology can run predictive models to make near-accurate predictions about lead quality or expected performance.
This use case has found its ground in manufacturing (forecasting inventory levels), e-commerce (product recommendations) and sales (personalized quotas to each sales rep).
With generative AI tools like Team-GPT, your organization can predict and handle quality-related issues before they can impact your bottom line.
Your brand can maintain high data quality standards that directly influence your decisions.
#5: Visualize Data
AI can also help your brand visualize data, such as interesting data patterns that the technology has found in your data sets.
There are AI-driven business intelligence tools like Luzmo that can help you build visual dashboards to help your decision-making and be able to present your findings to your team.
Luzmo’s AI chart generator lets you type a simple prompt and then receive interactive data visualization as a result, similar to Google Analytics 4 when you ask a question.
#6: Data Enrichment
Lastly, AI can be used in data analysis to enrich your existing data sets.
AI platforms like Team-GPT can supplement datasets with external data sets, such as economic indicators or social media trends.
What’s more, I’ve found Team-GPT to be particularly useful for imputing missing values based on existing data patterns.
For example, SEO teams can use AI technology to add parent categories to their keywords during their keyword research.
5 Examples of Organizations Using AI For Data Analysis
Here are the best 5 examples of companies using artificial intelligence for data analysis that our team was able to find:
#1: Sprint
After Sprint found out that they had a customer churn rate of 2.3%, which was way more than their competitors, they decided to turn to AI for a solution.
The brand used artificial intelligence to create a better customer experience by analyzing their data to get better at predicting outcomes.
The platform released a data solution where self-learning and predictive analytics would help find customers who were at risk of churn.
The algorithms were also providing personalized offers to some of the brand’s customers with the hope of retaining them.
In terms of results, the brand was able to reduce its customer churn by 10% and managed to increase its Net Promoter Score by 40%.
#2: Danone
Danone, a world-leading food company, implemented an AI-powered machine learning algorithm that can forecast product demand.
The corporation was running large-scale promotional campaigns and needed predictive insights into how many products each promotion would sell.
When it comes to results, the brand reported a 20% reduction in forecast error and a 30% reduction in lost sales.
The food company is now able to better prepare for seasonality and product demand during their promotions to further reduce operational errors and excessive inventory costs.
#3: Amazon
The e-commerce giant Amazon can personalize their product recommendations by collecting large data points of each consumer.
Amazon collects and analyzes all sorts of data about their customers, such as their past purchases and pages browsed on the website.
They then feed all of this raw data into their AI-powered recommendation system that is figuring out which product their consumers would be interested in.
The retailer has also hyper-personalized their home page to each consumer based on the data about product preferences they’ve collected so far.
Such hyper-personalization has allowed Amazon to stand out from its competitors with its customer experience, as only 15% of online brands believe that they’ve fully implemented personalization.
#4: A Client of Trigyn
I found a case study from Trigyn, an AI-powered analytics platform, that describes how they have transformed how their client interacts with their enterprise data.
The safety equipment manufacturing client needed real-time data access to support their consultants who needed data right away.
The solution that Trigyn offered was a user-friendly interface with natural language processing (NLP) capabilities, allowing their consultants to create on-demand analytics and visualizations without technical know-how.
As a result, the client saw a reduction in requests for ad-hoc reports, as their workers were able to receive instant access to the data they needed.
This technology has then received a high adoption rate throughout the organization.
#5: PayPal
PayPal was struggling with fraudulent activities in the past since they had to manually sift through all of them.
Instead of relying on manual investigation every time, which was both expensive and time-consuming, PayPal decided to give AI a chance.
This is why the brand started using the AI classification tool Simitily to flag potential fraudulent activities that require further investigation.
How it works now is if a credit card transaction is different from a customer’s spending pattern, the AI model would automatically flag it and raise it for manual inspection.
Best AI Tools to Analyze Data
Now that we’ve covered the main benefits and use cases of AI for data analysis, it’s time to explore the best software for analyzing data:
#1: Team-GPT
Team-GPT lets you collaborate with your data science team to develop AI models, analyze data at scale, and share actionable insights within a single AI platform.
Some of the clients we have worked with, such as Medesk, Polkastarter and Maersk have observed a 12x increase in productivity for tasks, such as:
- Predictive modelling.
- Data cleaning.
- Insights generation.
Our collaborative AI platform lets you choose the right AI model for your data tasks, such as OpenAI, LLaMA, Brad, Microsoft Azure, or the opportunity to bring your custom LLMs.
💡 You can work alongside your team inside of the platform by collaborating in chats, prompts, and threads.
It is also possible to create a clutter-free workspace with folders and sub-folders to organize your data team’s work.
That would also give you quick access to chats categorized by project or work type for easier access.
#2: Luzmo
Luzmo is an AI-powered business intelligence platform that lets you build client-facing data applications.
The platform is built for SaaS brands that are looking to add data visualizations to their platform within days.
You will also access AI-powered recommendations to pick the right visualizations of data and to make better decisions.
Here are some of Luzmo’s top features for data analysis:
- Sync real-time data by connecting to your preferred data source with the tool’s data connectors or open API.
- Build customer dashboards with a drag-and-drop interface. You will also receive chart suggestions.
- Generate instant customer insights with the tool’s instant chart creation.
#3: Rows
Rows lets you analyze, summarize and transform your data without having to use scripts or write code.
The tool, which is a Chrome extension, acts as your personal data analyst and can:
- Add tables: The tool can extract trends, generate pivot tables, and consolidate the data for you.
- Generate answers for you: You can ask the tool questions that you have regarding your data.
- Enrich your data sets with rich columns, which helps you improve your data analysis on Google Sheets.
- Create charts to help you turn the numbers into visual stories. You can ask the AI analyst to create various charts for you.
The data analysis software is powered by ChatGPT and can be used to classify and tag text in Google Sheets.
#4: AnswerRocket
Similar to Rows, AnswerRocket aims to be your go-to AI assistant for data analysis tasks.
The way it works is that you connect data from different sources and are then able to ask questions to receive actionable insights based on that data.
- Max, the AI data analyst: You can have a conversation with Max, the tool’s AI assistant, to understand more about your performance.
- Get answers to tough questions, such as ‘’What can I do about this problem’’ or ‘’Why have my sales been falling?’’
- Automate recurring analysis: Your business can receive daily, weekly, or monthly analysis upon a data refresh or a schedule to produce reports and presentations.
#5: Tableau
Tableau, a product of Salesforce, is an AI-powered business intelligence tool that was built for data scientists.
Your data analysis team will get access to advanced AI-powered predictions, be able to ask ‘’what-if’’ scenarios and do statistical modelling directly in the platform with R, Python, and MATLAB.
Here are some of Tableau’s top features for data analysis:
- Discover relevant metrics and get automated analytics in easy-to-understand language.
- Automatically detect drivers, trends, contributors, and outliers for the metrics that you are tracking inside of the platform.
- Get AI-powered insights that are personalized and contextual to the different employees in your organization.
Next Steps: Analyze Data At Scale Alongside Your Team on Team-GPT
You can analyze data alongside your team by building a customized version of ChatGPT on Team-GPT.
Our enterprise AI software lets your team analyze data sets by utilizing various AI models like ChatGPT, Claude, and Perplexity.
Apart from that, you can access:
- A comprehensive pre-made prompt library to create efficient workflows.
- Detailed usage analytics to track employee engagement.
- Enterprise-grade security ensures data privacy and the ability to host the platform on your servers.
Sign up for a demo of the platform today!
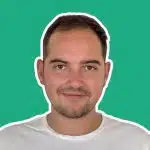
Iliya Valchanov
Iliya teaches 1.3M students on the topics of AI, data science, and machine learning. He is a serial entrepreneur, who has co-founded Team-GPT, 3veta, and 365 Data Science. Iliya’s latest project, Team-GPT is helping companies like Maersk, EY, Charles Schwab, Johns Hopkins University, Yale University, Columbia University adopt AI in the most private and secure way.